Regression
Abstract
Linear regression is a model for predicting the value of one dependent variable based on one or more independent variables. SmartPLS allows you to run linear regression models (i.e., single and multiple regression models).
Brief Description
As described by Hair, Black, Babin, and Anderson (2018), multiple regression analysis is a statistical technique that can be used to analyze the relationship between a single dependent (criterion) variable and several independent (predictor) variables (see also Backhaus, Erichson, Gensler, Weiber, & Weiber, 2021; Sarstedt & Mooi, 2019). The objective of multiple regression analysis is to use the independent variables whose values are known to predict the single dependent value selected by the researcher. Each independent variable is weighted by the regression analysis procedure to ensure maximal prediction from the set of independent variables. The weights denote the relative contribution of the independent variables to the overall prediction and facilitate interpretation as to the influence of each variable in making the prediction, although correlation among the independent variables complicates the interpretative process. Thus, it can equally achieve the objectives of prediction or explanation. The set of weighted independent variables forms the regression variate, a linear combination of the independent variables that best predicts the dependent variable. The regression variate, also referred to as the regression equation or regression model, is the most widely known example of a variate among the multivariate techniques.
The following figure shows a multiple regression model in SmartPLS. The software supports the estimation of single and multiple regression models (with and without intercept) and provides unstandardized and standardized regression coefficients.
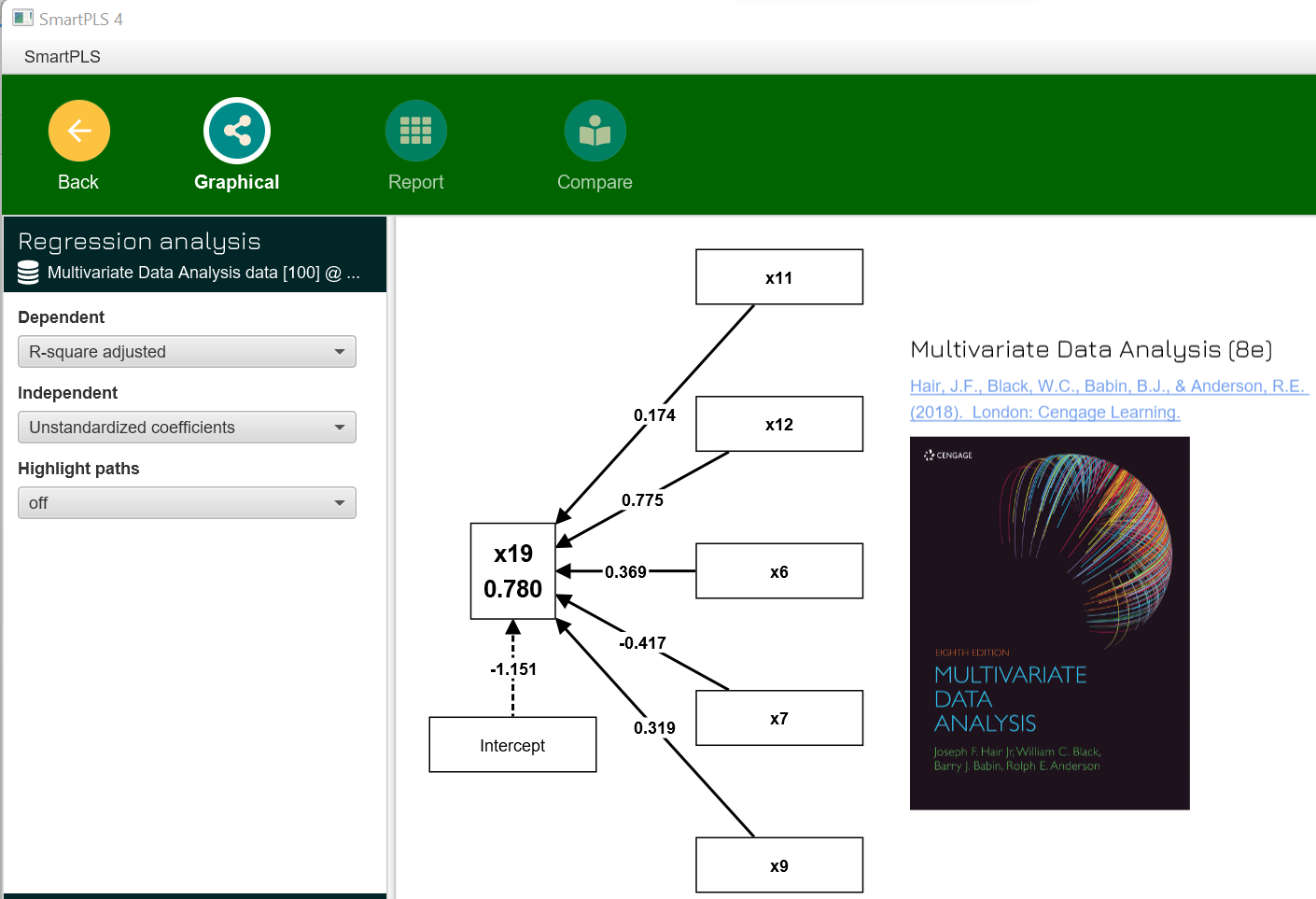
The SmartPLS results report for the regression analysis provides all the results to perform a systematic model evaluation according to generally accepted criteria. The following figure provides a results report example.
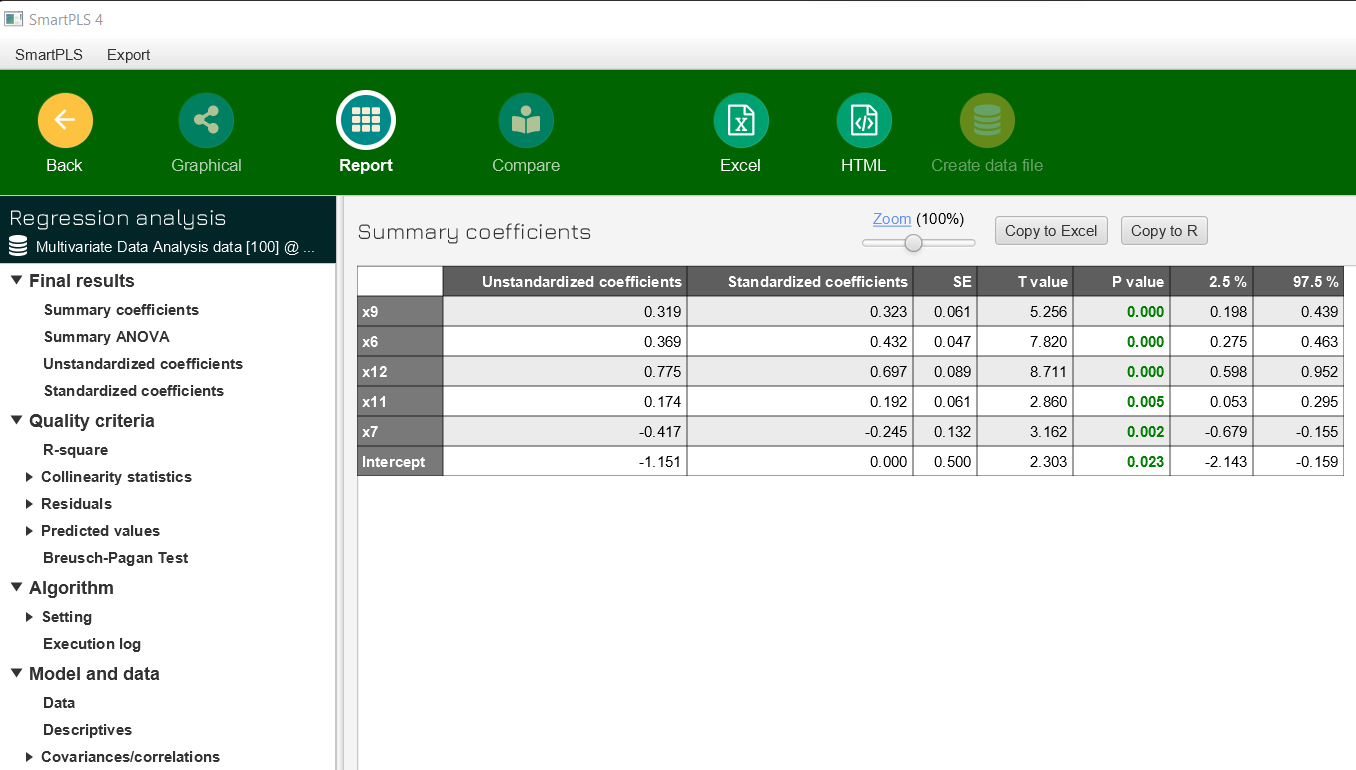
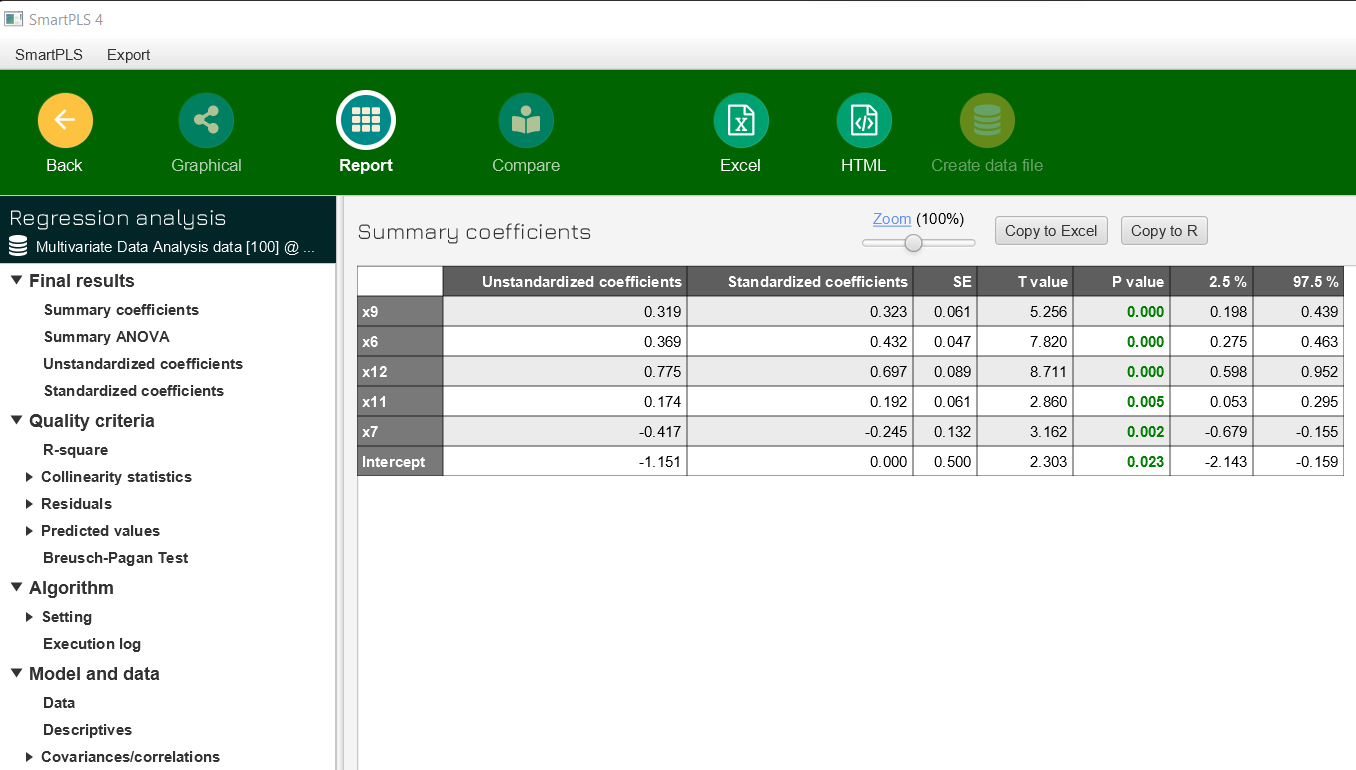
In addition, the SmartPLS report contains several graphical representations of regression model results. For example, the following figure displays the QQ-plot provided by SmartPLS.
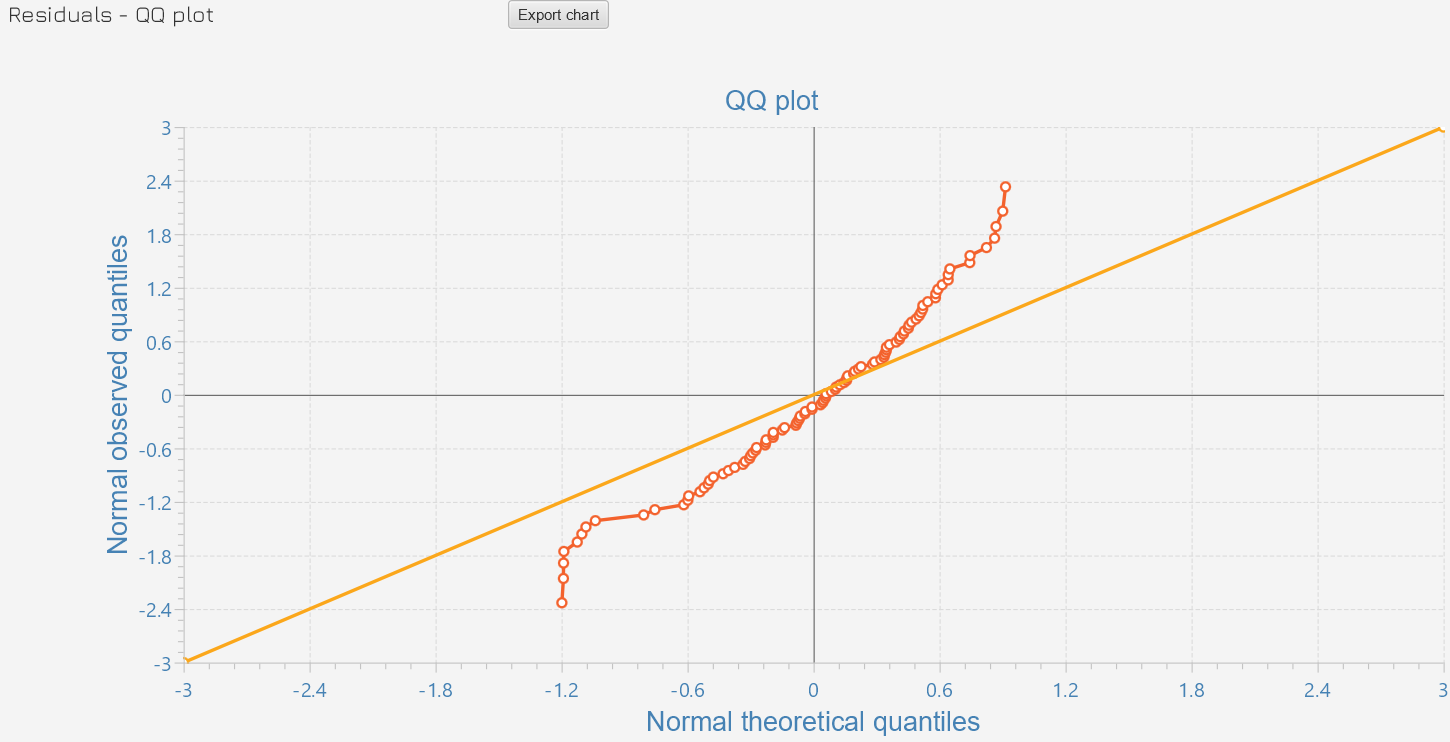
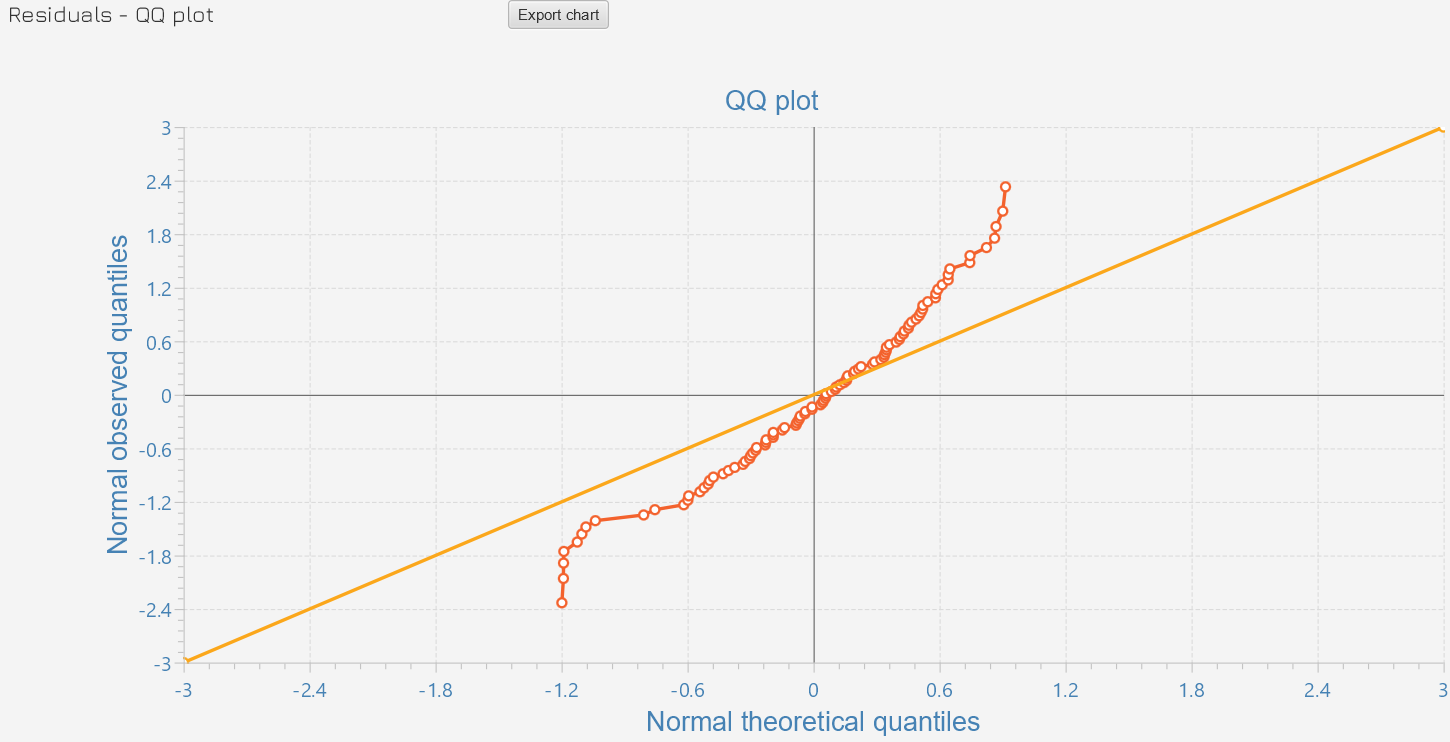
For SmartPLS, we provide sample projects for running regression analysis, including the Gaussian copula approach, logistic regression, and necessary condition analysis. Simply download, import, and run these examples in SmartPLS.
Regression Settings in SmartPLS
Test type
Specifies if a one-sided or two-sided significance test is conducted.
Significance level
Specifies the significance level of the test statistic.
Standard error type
As an alternative to the normal standard errors (HC0), users can select the heteroscedasticity consistent (HC) standard errors HC3 or HC4 in SmartPLS.
- Normal standard errors (HCO): Based on the original asymptotic or large sample robust, empirical, or "sandwich" estimator of the covariance matrix of the parameter estimates. The middle part of the sandwich contains squared OLS (ordinary least squares) or squared weighted WLS (weighted least squares) residuals.
- HC3: A modification of HC0 that approximates a jackknife estimator. Squared residuals are divided by the square of 1-h.
- HC4: A modification of HC0 that divides the squared residuals by 1-h to a power that varies according to h, N, and p, with an upper limit of 4.
References
- Backhaus, K., Erichson, B., Gensler, S., Weiber, R., and Weiber, T. (2021). Multivariate Analysis: An Application-Oriented Introduction. Gabler:Wiesbaden.
- Hair, J. F., Black, W. C., Babin, B. J., and Anderson, R. E. (2018). Multivariate Data Analysis (8 ed.). Cengage Learning: London.
- Sarstedt, M., and Mooi, E. A. (2019). A Concise Guide to Market Research: The Process, Data, and Methods Using IBM SPSS Statistics (3 ed.). Springer: Heidelberg et al.
- More literature ...
Cite correctly
Please always cite the use of SmartPLS!
Ringle, Christian M., Wende, Sven, & Becker, Jan-Michael. (2024). SmartPLS 4. Bönningstedt: SmartPLS. Retrieved from https://www.smartpls.com